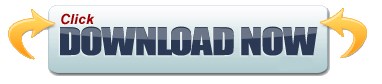
The performance of a fingerprint image matching algorithm relies critically on the quality of the input fingerprint images. After this process, the fixed-length vectors are fed into the first full connection layer.Īnil Jain, Sharath Pankanti, in The Essential Guide to Image Processing, 2009 23.9 FINGERPRINT ENHANCEMENT The final output is composed by concatenating three layers pooling results to generate a fixed-length semantic representation Km for input image of arbitrary size/scale, in which m is the number of sub-regions and K is the number of the features maps in the last convolutional layers. In each spatial sub-region, SPP-net is used to pool the responses of each convolutional kernel. Three layers pyramids are used to extract high-level semantic features, in which the divided sub-regions are set, respectively, n × n as 1 × 1, 2 × 2 and 4 × 4. SPP-net is viewed as convolution operation, where the size/scale of sliding window is win =⌈ a/ n⌉ and the stride is str =⌊ a/ n⌋, where ⌈.⌉ and ⌊.⌋ are ceiling operator and floor operator, respectively.
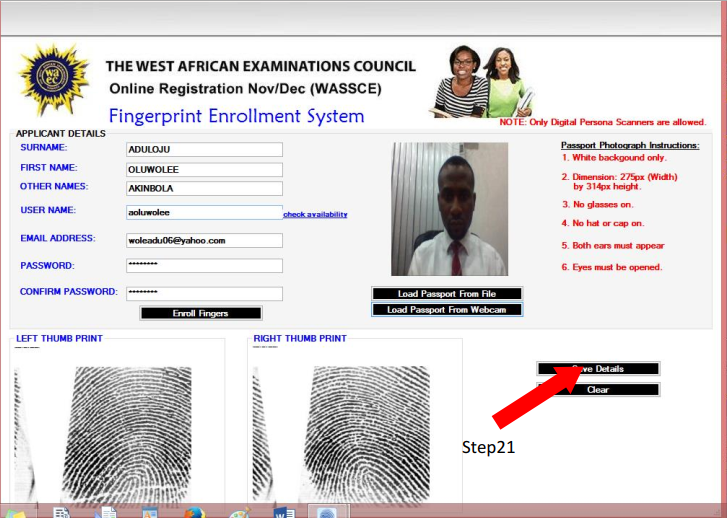
Suppose the size of the last convolutional layer output image (feature map) is a × a, each feature map is divided into n × n blocks. Afterwards, to eliminate the image size problem, the spatial pyramid layer has been added between the last convolution layer and the first full face layer. It contains two full connection layers, and a classification layer. 8, in our scheme, following five successive convolutional layers and two successive general pooling layers, a spatial pyramid pooling layer is installed. Features maps of the last convolutional layers are partitioned into sizes proportional to sub-regions, so the number of sub-regions is fixed for images of an arbitrary size/scale. The SPP-net keep spatial information by pooling in the local spatial sub-regions. Therefore, we establish a network layer structure based Spatial Pyramid Pooling network (SPP-net). To achieve this goal, a fixed-length vector set is necessary for the classifiers or full connection layers.

Our scheme attempts to learn multiscale high-level features, so as to capture detail spatial information. The scale of fingerprints images is various, due to different fingerprint acquisition devices different scale contains different detail information of the images.
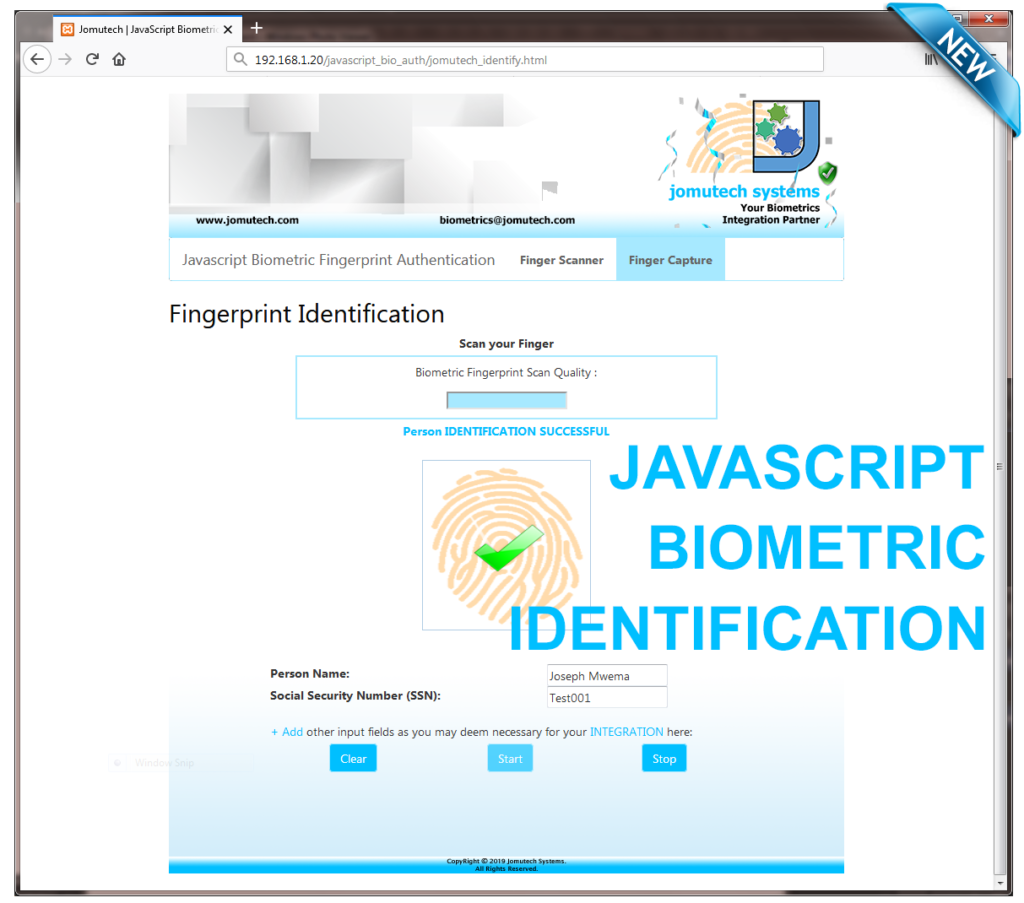
Sheng Wu, in Advances in Computers, 2021 3.2.2 The construction of SPP-net deep feature vector
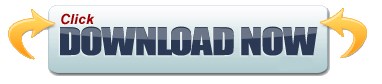